Duncan is no stranger to pushing boundaries. When he’s not working with our clients and their digital products and platforms, he can be found experimenting with new technologies.
A great example is his pioneering work with QR codes. Read about that here and learn more about how he found himself fielding dozens of requests from all around the world on Christmas Day. Duncan was also the chief architect behind the Kyan jukebox, before opening up the application to the wider tech team for further development. You can read more about that here.
This time, we’re talking artificial intelligence. For the coming weeks, Duncan has kindly lent us his time and his brain to share his thoughts and feelings on AI, and more specifically Generative AI and Stable Diffusion. Later in the month, we’ll have a deep dive Dev Talk and accompanying video to share with you. But to kick it all off, we spent ten minutes talking with Duncan as an intro to his experimentation with AI image generation.
Amy: Hi Duncan. Let’s start from the top. What piqued your interest when it came to AI image generation?
Duncan: Like many people, I started to notice the rise of AI image generation purely through some of the weird and wonderful creations that were being posted on the web. And naturally, I wanted to know more.
Much of this was people changing their profile pictures to look like famous fictional figures such as Iron Man or Robocop. But it was the high fidelity of these images that really got me interested, and I found myself asking “Okay, what is this?”. It really seemed that the images I was seeing were getting better and better by the week.
I quickly came to understand that something called Stable Diffusion was the particular AI model behind a lot of what I was seeing. They’re certainly not the only developers doing this kind of work, but they have been the only ones to make their technology public and accessible – essentially open source.
For those who don’t know, you simply give a text input (the prompt), which is a description of what you’d like to see, and the model’s output is an AI-generated image that attempts to match your description.
I find Stable Diffusion particularly interesting not only as an AI model but a business model too. It’s a collaboration between interested parties with the intention of open sourcing from the start. In fact it was designed so it could run on consumer hardware.
It clearly costs a lot of money to develop and run, and must involve a lot of other technologies and services. I think it’s brilliant that at some point during their work, they decided to give it to the public and say “Now, what can you do with this?” So that’s one of the reasons why you’re seeing pictures of friends looking like Star Wars characters, and I find that fascinating.
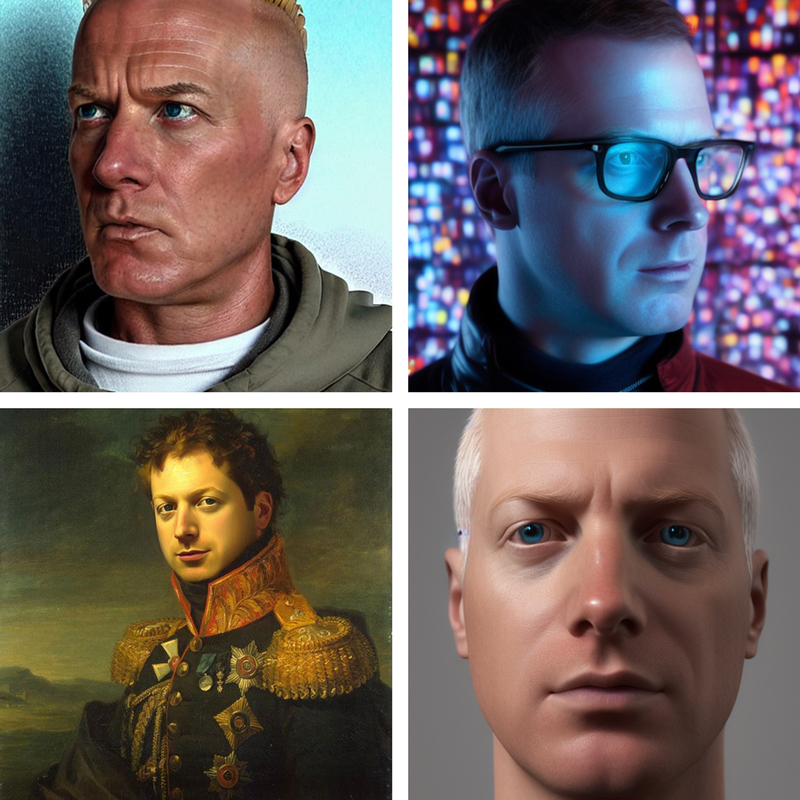
A: So let’s park this excitement for a moment and look on the other side of the coin. People are creating digital likenesses of themselves based upon established art styles or well-known characters, alive or dead. Is there an ethical line here?
D: There is an ethical line and I think it’s quite complicated. The big question, for me at least, is around ownership. I’m not talking about the ownership of the generated images themselves, but the ownership in the sense of copyright. Creating a piece in the style of Van Gogh is one thing, but what about creating a piece in the style of an emerging artist who doesn’t have the same legal protection or powerful estate?
It’s ultimately very easy to create a piece of art in the style of a famous illustrator with just a few descriptive text prompts. If that’s a self-supporting artist who relies on the sale of their work to make a living, that’s certainly an ethical issue as you’re nabbing their work, not to mention the harm it’ll do for their web discovery (imagine the SEO nightmare of losing your own work amongst results that show hundreds of AI-generated copies).
It's a really interesting conversation and I can see it from two different angles. Primarily, I see it from the artist's point of view and how this can be a very real threat. But secondly, this stuff isn't going to go away any time soon, and the technology will only ever get better and better. That's just how these technologies work. The same way ‘home taping’ threatened radio and TV, and even further back, photography being a threat to painters. Everyone has to figure out how they can fix it together, because otherwise you get left behind. I don’t have the answer here but I think it’s on all parties to find a workable solution.
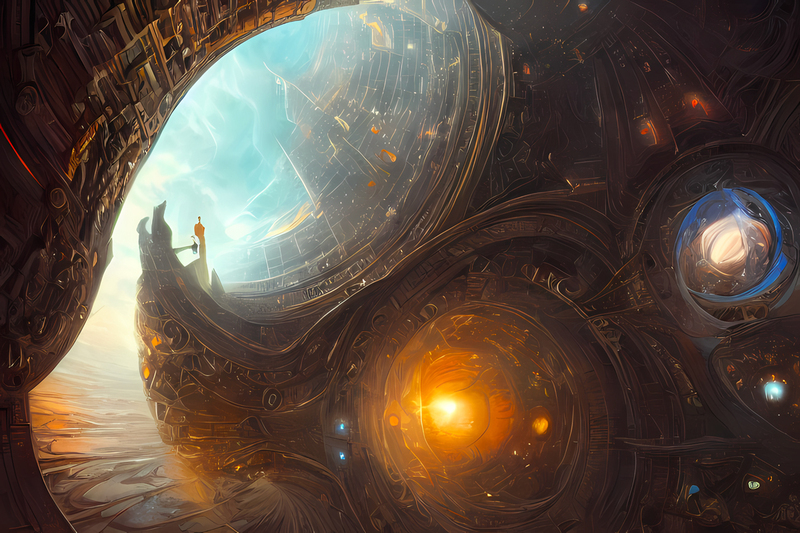
A: Can you share with us what you’ll be talking about in your upcoming Dev Talk?
D: The idea started when I asked myself if I could train a model to draw illustrations like a particular artist. The short answer was, yes, I could. And it was really easy. I ran a couple of examples, and some artists were easier than others. For example, an illustrator that uses bold colours and simple lines is easier to fake than one that uses a more complex and detailed approach.
Ultimately, I trained this model with five or ten fairly generic images and I asked the model “Can you draw me a bunch of flowers on a table in the style of this artist?” Considering the results, I believe that the artist would be able to recognise their own style but also identify that it’s not their original work. But for me, as a human with an untrained eye who isn’t familiar with the artist's work, I’d have a hard time telling if it was real or not.
Overall, I found this pretty mind blowing, because really, I didn't do anything. I didn't do anything technical, didn't tweak anything, didn't spend hours regenerating stuff until something looked nice. It was the first output.
So that’s the basic image description stuff. But when it comes to fictional characters and profile pictures, I found that it wasn’t quite so easy. In many cases, the final piece (or at least, the piece that the user has settled upon) is so high fidelity. But as I started to explore the software, I realised that I didn’t always get the best result first time. Sometimes it could be as much as the 100th time.
The model is using other bits of technology within this generative AI to fine tune it and make it nicer (higher fidelity) and make it look much better (more accurate). The technical term here is ‘in-painting or out-painting or image-2-image’, and these are mechanisms for taking an existing image and improving it, or taking a small part of an image and drawing it outwards, and it’s this part of the model that I want to explore in my talk.
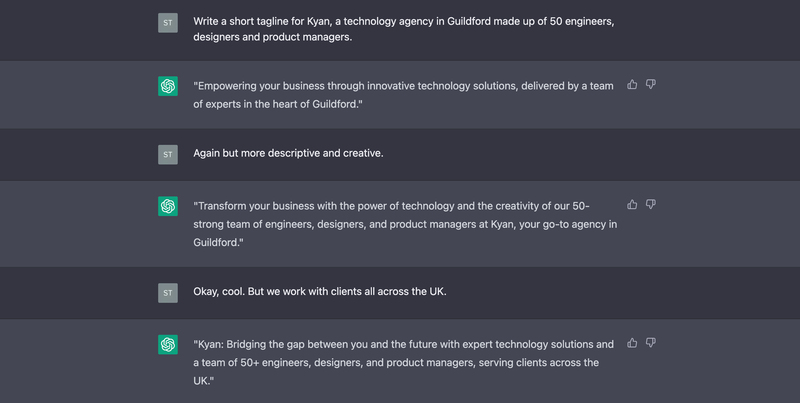
A: Let’s talk a little about ChatGPT. As a marketer, I’ve been experimenting with how I can use it to complement my work. Have you tried using it to enhance the work that you do? And are you worried about it in the same way that artists may be worried about AI image gen?
D: You know, I fear typing into it and asking it to write some software for me and thinking that's better than I could ever write! But I don’t think based on what I’ve seen so far, it could replace humans when it comes to engineering, coding and development. It’s not creative enough, it doesn’t understand nuance, or the wider context of a project. And in code and development, I think that’s particularly important for writing good software.
Maybe that will get better over time. But for now, as an engineer, there are still things I don’t know, so like many others, I spend a degree of time Googling and looking at StackOverflow. I don’t know any developers who don’t do this, but the problem is it’s time consuming, there are a million unanswered forum threads, and technology changes quickly. This is where I think ChatGPT can be purposeful – it can construct an answer quickly. Whether it’s right or wrong remains to be seen, but as I keep saying, it will learn and it will get better.
A: The continuing theme here is that “It will get better and better”. In the near future, that’s exciting. In the long-term, that could be quite a worrying reality to some. Does AI at this level need some kind of gatekeeping?
D: I definitely think that there should be some kind of moderation. I believe it should be free, but I don’t believe we should be free to do whatever we like with it. There needs to be steps to try and make it all a bit safer, because the image generation model is an absolute minefield when you consider what the minority of horrible people could be capable of. You can make all sorts of horrible imagery in terms of discrediting people or in terms of generating images that cross moral, ethical and even legal boundaries.
I think there needs to be something figured out around that, and around stealing people's work and taking away people's work. It feels like it's a bit too easy at the moment and it looks like steps are being made. When I started looking into Stable Diffusion, they were already discussing these issues at version 1.5, and now they’re at version 2.1, so they’re clearly putting the work in (for comparison, version 1.0 was considered ‘not safe for work’).
What I do know is that they’ve redacted a lot of artists’ names so they can’t be used in the prompt. So if you do try to create an image in the illustrative style of a particular artist, maybe that won’t work. They’ve also discussed digitally watermarking the imagery, but I’m not entirely sure what they mean by that or how that can actually work. Maybe it’s not a watermark in the traditional sense. So that remains to be seen.
We’ll be sharing Duncan’s Dev Talk in the coming weeks, followed by several more pieces of content covering generative AI tools and models. Keep your eyes on Kyan social channels!
Previously from Duncan:
Using Semantic Release and Github Actions to build releases
Using Swift/SwiftUI to build a modern macOS Menu Bar app
We are Kyan, a technology agency powered by people.